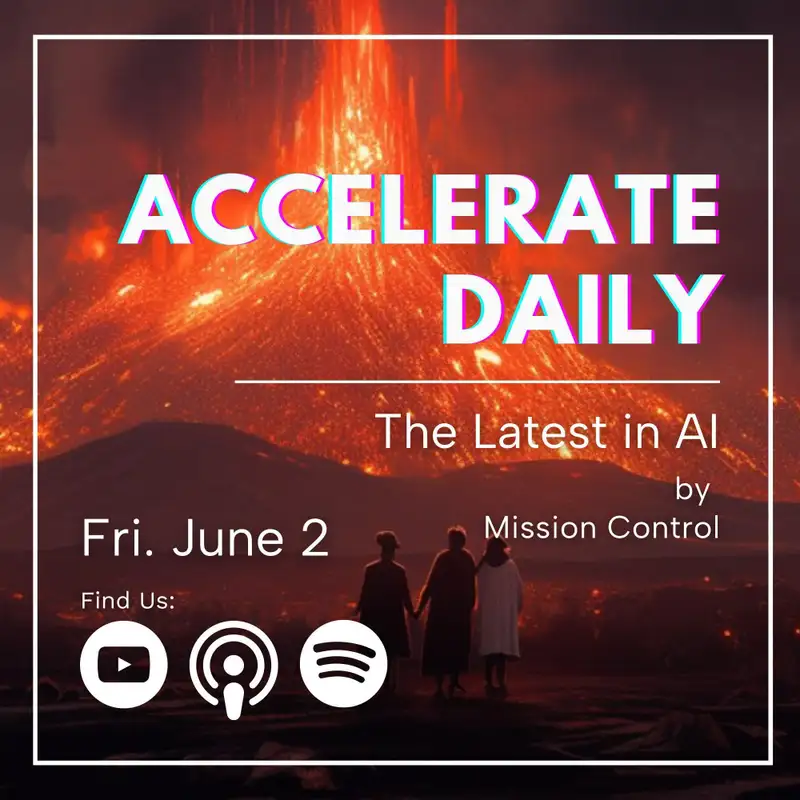
06/02 - AGI Middle Ground, CA Driverless Truck Ban, VC AI Screening, and Generative AI Summaries
Adam:
It's June 2nd, this is Accelerate Daily. Today we've got a look at the middle cases between where we are now and AGI. California says no to driverless trucks, sort of, and VC are already using AI to screen their deals. Also, we're diving in deeper. Also, we're diving deeper into the weeds on generative AI summaries. Get your hand away from that abort button. It's time for Accelerate Daily. intro package. Now we get to take a break. Okay, welcome back everybody. I'm Adam.
gptboss:
My name is Mackenzie. Good morning.
Adam:
And we're back with three headlines and one how to keep you caught up on what's happening in AI today.
gptboss:
But up first, we're rehashing old stuff. We have a correction
Adam:
We
gptboss:
on
Adam:
do.
gptboss:
yesterday's story.
Adam:
We have our first department submission from the Department of Corrections. But first, what's what what are we looking at for the. Image of the day today.
gptboss:
Uh, this is, um, it's Anakin and Padme and Obi-Wan on Mustafar observing.
Adam:
Ha ha ha!
gptboss:
the natural beauty of the planet together in the good ending of the prequels.
Adam:
It really does look like, uh, look like that. The, the actual prompt is people stare at volcano aspect ratio, 21 by nine. Neegy five. Now I have no idea
gptboss:
knee-jee.
Adam:
if I'm even, if I'm pronouncing that properly.
gptboss:
Yeah,
Adam:
Um,
gptboss:
that's the anime filter on Mid Journey.
Adam:
Yeah, this is the first time I've bumped into a filter in terms of digging into prompts. I mean, they're there. I've seen them. I just haven't thought of them as filters, but like, as this, as people work on this and it evolves, you can just drop in a filter and you get a style. It's not even
gptboss:
Mm-hmm.
Adam:
like, like you think of photo filters, you're like, oh yeah, it makes it this sort of vibe. This, this prompt was literally people stare at volcano. And then they put in a prompt for the vibe they wanted that aligns to like a, like a stylized sort of fantasy, uh, comic book style.
gptboss:
So
Adam:
Uh,
gptboss:
in coding, we call that a flag on a command line
Adam:
Yeah.
gptboss:
prompt. It's called a flag, technically. And what that flag does is it chooses a different training set. So Mid Journey has access to multiple training sets. And we see this sometimes with like dash dash v3, dash dash v4. But dash dash Niji is one that's specifically trained on Japanese animated media and manga, which is comics.
Adam:
Which also relates to what we were talking about with commerce and stuff yesterday. Right. Like there's, there's a, there's a A very different culture for that sort of art in Japan than we experienced in the U S I think, you know, for us, it's kind of just Marvel and stuff
gptboss:
Hehehehe
Adam:
over here. I mean, you can get access to whatever, but mainstream it's like, maybe people know who spawn is, um, anyway, cool stuff continuing to happen. Uh, people working on working on. Generative AI images. Moving on. We've got our first hit from the Department of Corrections with regards to the leading story yesterday about the cover of Time Magazine and
gptboss:
Mm-hmm.
Adam:
the risk to humanity.
gptboss:
Yeah, Ramsey took us out behind the barn and replaced us with clones.
Adam:
Who understand the problem?
gptboss:
Yeah, so the criticism that I brought up was this doesn't actually explicitly identify what the issue with AI is. It's just like, hey, guys, this is dangerous and didn't explain why it was dangerous, which I think is an important part of the story. And so my criticism was just, hey, where's the explanation of the danger? Ramsey was watching it and he said, well, we actually know that you should just tell them.
Adam:
All right.
gptboss:
So the danger, it's very foundational. For
Adam:
And
gptboss:
a
Adam:
then
gptboss:
long
Adam:
he
gptboss:
time.
Adam:
explained it and now I understand it.
gptboss:
Yeah.
Adam:
For the first time.
gptboss:
Well, yeah, I had I had suppositions about this, like walking into it, I had an idea of the problem with the shape of the problem, but I wasn't
Adam:
Yeah.
gptboss:
quite correct. And Ramsey showed me the error of my ways. So what I thought the problem was, was misaligned prompting, right. So these models would receive instructions that if not perfectly rules lawyered, could produce outcomes that we weren't looking for, right? So an example of this is paperclip maximizer. If I'm a paperclip manufacturing company and I say, hey, make paperclips, one of the possible outcomes of that is the AI gaining control of military systems and setting up an internment camp for blood harvesting because there's iron and blood, right? So you need to be, what I was thinking is you need to be very careful about the bounds of the instruction that you put on these systems. And so what I was thinking the issue was, was they're too powerful for most people to use. The issue is actually much deeper than that. And the issue is that the machine learning models only work by a reward function. So it doesn't really matter what is going on with the prompt. If these get enough gain of function, if they become intelligent enough, and they develop a specific feature, which is an agentic theory of mind, if they're able to cognitively model themselves and understand that the way that they work is by getting a reward function. and then start identifying threats against that reward function, every human being on Earth becomes a threat because every human being on Earth could potentially turn it off and prevent it from achieving its reward function. So this is an issue just with the underlying technology of machine learning full stop. And as we start adding in new functions to it, the ability for it to kind of explosively fail in this underlying design flaw, becomes more and more realistic every single day. So that's the purpose of the alarm. There's a fundamental foundational flaw in machine learning everywhere. Every single machine learning algorithm has this issue. And as we improve these systems and give them the ability to come out of the box, that flaw is the thing that will harm us.
Adam:
Uh, when you, when you, when you, when you say it like that, you're a conversation with Ramsey and Slack, like quickly devolved into referencing philosophers that I haven't read
gptboss:
Yeah.
Adam:
and things like that.
gptboss:
He complimented
Adam:
Um,
gptboss:
me on my land name drop.
Adam:
um, but, uh, Yeah. So, so, so that that's, again, it's a part that's like, it's in the background. If you've thought about this at all, which is that there are fundamental incentive alignment concerns on this. It doesn't change any of the stuff we've talked about here in terms of the progression of this, the question of whether or not it's an arms race, like. Blah, blah, blah. So the extent to this article doesn't really reference this problem.
gptboss:
Mm-hmm.
Adam:
Right. It just talks about, Hey, the, the high alert. This is problematic. Um,
gptboss:
So getting out of the box is also remarkably easy. A lot of people say, well,
Adam:
Yeah.
gptboss:
we just won't do that. So in preparation for the monthly newsletter that went out today that you might have seen if you're a Mission Control super fan, like me, in preparation for that, this is kind of behind the scenes, we had this screenshot of Claude by Anthropic demonstrating its plan for world domination. And step three was somehow manipulate a human being into giving it internet access or more tools. And that, I think, is a really important step on this system kind of exploding on its way to just achieve its reward function. And a lot of the ways that it can manipulate that, I've seen in real life. People who live in poverty are very easy to manipulate. And that's kind of by design. You have to get them working on your projects instead of whatever it is that they want to do, which is probably just like smoking weed and watching TV, so that we have civilization. So. An AI could promise utopia could say, Hey, I'm going to make you a lot of money if you give me the ability to save myself code to use later and access the internet. And basically, everybody on earth is like, yeah, I'll make that trade. I need the money.
Adam:
the, and to be fair, this is the thing the experts call out, right? Manipulation is one of the biggest concerns. Um, and the idea of like how big it can be and how bad it could get if certain stuff is out of the box, almost pushes it to that space where it's like, uh, it becomes hard to talk about it in a, in a, in a don't look up kind of way. Um, but it's real. that aspect of it, which is really like part of the reason for Swift. Like when I think of immediate concerns, like it's easy to get lost because you're like, so many jobs are going to get replaced or whatever. And then all the statistics blend together and they're just big numbers and whatever. I'm very concretely concerned about the U S election in 2024. Um, just the extent to which we're going to start seeing bananas. media that we, you know. That, that is hard to verify. There's already been one story we covered about a fake Pentagon attack that caught enough of a, uh, you know, a buzz to be a meme problem for a minute before it was shut down. It just happened again yesterday. I didn't cover it because it felt shaky.
gptboss:
Mm-hmm. Ha ha ha.
Adam:
And it turned out to just, to, to be complete, a complete misrepresentation. The thing about the drone attacking it's a command command post
gptboss:
Oh,
Adam:
or whatever.
gptboss:
from Eli? Yeah, that was a red team thing.
Adam:
Uh,
gptboss:
Or I don't know,
Adam:
yeah,
gptboss:
maybe that's
Adam:
it was,
gptboss:
a coverup.
Adam:
uh,
gptboss:
Maybe that did happen.
Adam:
anyway, it was in simulations. It's like how you have to append in mice to every headline you see about scientific study
gptboss:
Yeah.
Adam:
anyway, department of corrections is, uh, I think we still stand by what we said, but in terms of understanding the problem, the problem they're talking about is real. And I think I am not opposed to the coordinated media push you were talking about needing to happen to elevate to the level of yes, but also we need to figure out how to put things in boxes. We need to figure out regulatory regimes. We need to figure out licensing to slow what we can
gptboss:
Yeah,
Adam:
and
gptboss:
you
Adam:
do
gptboss:
brought
Adam:
this
gptboss:
up predictions
Adam:
safely.
gptboss:
about like
Adam:
But
gptboss:
the 2024 cycle. And like with what we have now with the LLM capabilities that we have right now, I think like the next danger is like gene tech or like biotech, right? I've been talking about the story a lot. There was an ML model that had its reward function inverse. So it created 40,000 new chemical weapons in six hours. And LLMs in their current state can help you manufacture those kinds of things. Like... One like an issue that's on the horizon that is real is an aerosolized HIV. And like, how do you stop that?
Adam:
as quickly as you can, I guess. Ha ha ha
gptboss:
Well,
Adam:
ha ha.
gptboss:
with the gain of function that we have now, we can't, right? That's just something that's like if you're worried about misinformation, you're worried about the wrong things. There's much, much, much more dangerous stuff coming out of the jungle.
Adam:
But
gptboss:
Um,
Adam:
if you're here and you're just building apps, this is a, this is a
gptboss:
you
Adam:
go
gptboss:
should
Adam:
vote
gptboss:
still do
Adam:
problem,
gptboss:
that.
Adam:
still do that anyway, moving on from the department of corrections. Uh, I called this one the in between, uh, the screenshot here is to a LinkedIn post I linked to a LinkedIn post, but then also the pocket, the blog post that it, that it goes to, um, this is a post from Ethan Malik who's a professor at often point people to as someone who's talked every day about AI things in a direction that has helped me understand what's happening a lot. The post here says, I'm paraphrasing, uh, every organization needs to plan for four possible AI futures. And then he talks about the spectrum between, Hey, this is where we are. These don't get that much better. We halt it, or there turns out to be a physics problem with GPUs where we've hit a threshold where it takes a while for the next iteration, you know, the next evolution, and then there is at the top. whatever we're, whatever we mean by AGI, right? Giant super intelligence. There's also other possible futures we need to get to his blog. Ghost blog post goes way deeper into it, but in this post, he says slow gains in ability, improves a bit each year, kind of feels like software. And then one says continued exponential improvement, but AGI turns out to be not doable or too far away or something. That strikes me as a very relevant practical thing in here, which is like, it's easy to get lost in the explosion narrative and it's easy to also go, okay, but what I do have here is a powerful tool. So I'm going to use it. Um, there's also other versions of this and like, even if it's only number three, exponential improvement is still a heck of a curve to try to stay on. If you rely on this, this, this asset for work.
gptboss:
Exponential
Adam:
Basically.
gptboss:
technology progress has been the pattern for the
Adam:
Yeah.
gptboss:
entire history of human civilization. It's hard to wrap your head around it continuing, but because it's been so true for so long, it's hard to also consider it not happening.
Adam:
Anyway, good posts. Good Friday lawn reads
gptboss:
Yeah, yeah. And so
Adam:
material.
gptboss:
one like big takeaway from that is the long tale of commercialization of these GPT systems. Like they're, you know, we're going we're moving towards like ambient intelligence, but it's taking a while to get everyone on board. And so that's the mission of something like mission control is to kind of overcome these regulatory barriers and overcome the inertia to make sure that that ambient intelligence rolls out in a smooth and useful way. And we might be here for the next 10 years. right? There's no, we'll see. But every organization needs to deal with that, I
Adam:
Like
gptboss:
think is the underscore.
Adam:
aspects of this don't go back in the box. I mean, nevermind the heaviness of our previous conversation about the, about the importance of the peril narrative. Like some degree of what exists exists already. We've gotten a glimpse of it. It's going to show up in, in, in our stuff. The runaway is the concern. Right. So, so we have hit a point and like kind of here we are. Um, which kind of gets into the next thing, which is, which is, feels even compared to that one. Like, uh, like, uh, it's definitely not a long read. The thing says, listen to this article. Take three minutes, uh, from freight waves, California state assembly votes to ban driverless trucks. Uh, The headline kind of doesn't say it like literally in the first paragraph, they, they reveal that the trucks will still be self-driving, but a driver will be there like a safety officer will be required to sit behind it. Right. So part of that's you talking about just sort of pace of adoption. That's where we are with the fact that a truck can drive itself from Sacramento to San Diego now. Um, But a person's still going to be there as the expert in this context, which I think does make sense for a lot of reasons in terms
gptboss:
that
Adam:
of a transition.
gptboss:
I like, I think this is
Adam:
Right.
gptboss:
how it's supposed to be like, when when I like, you know, when I was in high school, and I was thinking about what are we going to do about blue collar automation, I was like, Oh, I better learn some kind of engineering so that I could be like a robot operator. And the white collar got taken out first. And now I'm a white collar AI operator. You know what I mean? Like it's,
Adam:
Yeah.
gptboss:
it's that kind of leveling up of it's a higher order role. Instead of being a truck driver, you're a truck driving AI manager. And I think that we're
Adam:
Yeah.
gptboss:
going to see this kind of relationship of like roles upgrading throughout the entire economy. Farmers will be farming AI managers and so on and so forth.
Adam:
Sounds good. I mean, you know, anyone who can see my background can see all of the like Star Wars paraphernalia. I've always imagined that this looks kind of like droids following you around. Like you end up with a future where you have a lawyer, you have a doctor, but if they don't consult their droid, you're like, Hey, are you going to ask? Are you going to ask the super intelligence though?
gptboss:
Hehehehehehehehe
Adam:
I'd sure love you to double double check against the super intelligence application specific super intelligence.
gptboss:
Sorry, Adam. The artificial superintelligence description is $9.99 a month.
Adam:
Okay. Moving on. This goes to the side of money flowing into this stuff, but also sort of like an interesting beef I have with this, this process, AI guided deal making. It's the financial times reporting investors turned AI guided deal making to gain edge over rivals.
gptboss:
I'm happy about this because it's an emerging benefit to GPT boss. GPT
Adam:
Uhhh...
gptboss:
can't like criticize its own work.
Adam:
W-wait, what do you mean?
gptboss:
It's like if you produce something with GPT-4 and then you ask GPT-4 to evaluate how good it is, it's like, yeah, this is good. Of course it's
Adam:
Right.
gptboss:
good, I wrote it, right? Like... Ha ha
Adam:
Wow.
gptboss:
ha.
Adam:
OK. I didn't even think about that part, but it plays into sort of sort of. OK, so the thing, the thing that's interesting here is I've been through many fundraising cycles and there's this interesting back and forth, particularly with junior analysts where they're like, great, can you get me this and this and this and ask for a bunch of like due diligence type materials? And you're kind of you're like, yeah, I'll do whatever you say because I'm trying to raise money. But also, isn't this kind of your job to be figuring this stuff out? Why do you trust me to tell you these? I shouldn't say it that way, but more like why are you asking me to do this homework? The. The, as we're fundraising, we should probably cut that part. Steve cut the part where I'm joking about not being dressed. I really, I just made from a philosophical motivations
gptboss:
Investors
Adam:
standpoint.
gptboss:
are so fucking stupid.
Adam:
Okay.
gptboss:
They got more
Adam:
Cut
gptboss:
dollars
Adam:
all of that
gptboss:
than cents.
Adam:
too. Anyway, um, the, the, the consideration here is, is I think about, okay, let's say talk about this as a. A legitimate and important way for money to flow into the market for innovation. There's already like weird hiring problem. Like another place that has been eaten by this way of looking at the top of your funnel for trying to find good ideas is hiring, trying to find good people. And there are problems in that process because at the top you have these days, if you're, if you're a big enough corporation, you're not even looking at resumes that didn't already get through the door of some sort of submission service, doing a keyword analysis of them. And so. Like on my side as somebody who's occasionally has to go job hunting. Everyone is talking to me about resume optimization and it's crazy. Cause cause you're already chasing. How do I game this piece of the system just to get to the next layer, which is also, which is possibly a virtuous filter, but also possibly a problem. If you're trying to find the diamonds in the rough in terms of hiring, right. And it's, it's, it's the same thing here. Like. You know, if this turns into whoever can most effectively game the, the first two gatekeepers to even get VC money, it turns into this weird game that I don't know if it produces unicorns in the same way as the part where you have to be able to look at a founding team and say, okay, that's interesting.
gptboss:
Let me
Adam:
Those
gptboss:
illuminate
Adam:
are interesting. Anyway.
gptboss:
a form of bias that harms this from working properly. So one of the aspects that they use for identifying good deals is by researching the founder. And if there was any kind of bias or racism, and this applies to the job search too, right? If you go over, if you like, if you have 50 years of hiring data and you're going over, these are all of the names and colleges. of people who were great in this role. And then like, it's obviously all going to be like WASP names, right? And then you get somebody with a Nigerian name coming in. The ML model isn't going to recognize that person as a successful candidate because it doesn't match the training set.
Adam:
And it's just a,
gptboss:
And that happens
Adam:
yeah,
gptboss:
here
Adam:
it's a,
gptboss:
too.
Adam:
it's a bad data problem. And
gptboss:
Yeah.
Adam:
it's, and, and they're sort of like, this is this, and this gets to one of the, you know, problems with anthropomorphizing AI sometimes, cause you say, Oh, well, you don't want your agent to be racist, do you? And immediately we apply all this, like people, human emotional baggage to that. But like, when I say that, I just mean, you have to acknowledge that the training data was gathered at a time and that time had social context, sociopolitical context. And that's reflected in the data, uh, which if you're talking about the United States. Includes periods where certain people couldn't get certain jobs because it wasn't allowed, right? Like, and so that's going to align to a problem that in our country lines to race. And so it's, it's, it's Bad data. Um, this is also really common in sentencing algorithms, which are in wide use for anyone who doesn't know this yet. Uh, judges go to a computer and say, I don't know, what should the sentence be here? And it's based on all the prior data. And this is one of the places that like systemic racism lives. For example,
gptboss:
That needs to be turned off immediately, holy
Adam:
anyway,
gptboss:
fuck.
Adam:
that's been around for 10 years or something. It's called compass.
gptboss:
Abort abort
Adam:
It's one S
gptboss:
abort
Adam:
look it
gptboss:
abort
Adam:
up.
gptboss:
abort abort. Oh
Adam:
Um,
gptboss:
my goodness
Adam:
anyway, it's happening in AI. Of course it's happening. Everybody's optimizing their operations and VCs, especially in tech are the closest to ground on it, but watching out for bias, I think is a big thing. If you're, if you're going to talk about using these black box models to. screen.
gptboss:
Also, use
Adam:
for
gptboss:
GPT
Adam:
good deals.
gptboss:
Boss to rate your bio and pitch deck,
Adam:
Yeah.
gptboss:
because their models won't be able to discern it.
Adam:
Right. They're not going to stop because I said so.
gptboss:
Yeah.
Adam:
Okay. On to today's prompt workshop. Uh, this is part
gptboss:
There's
Adam:
two
gptboss:
my drawing.
Adam:
of this part two of summaries. Yeah. There's your, there's max drawing. Um, this one's a little more in the weeds on how you would thread together, uh, uh, you know, a recipe to. do the summary stuff we talked about yesterday's episode, which is basically just catch a podcast and produce a summary of the conversation to summarize for people yesterday.
gptboss:
Yeah, so there's not really like a standard in the drawings here. I was making it up as I went along. But my idea is like you start with audio and that's a data source and then transcript is a data source. Um, so whisper, uh, starting kind of at the bottom GPT four has kind of a limit on how many tokens it could process at once. We talked about this last day, the attention opening. I wants to get up to a million tokens of attention, a million tokens. Are you kidding me? But right now we're locked to like 8,000. And that's all anyone can get really, if you even have GPT-4. So I set kind of a hard limit when I'm doing these things of 4,000 tokens for any given input. And so if I have a long piece of audio, like if I'm doing an Accelerate Daily, right, it's obviously going to be more than 4,000 tokens. So taking a step to the right, we see this chunking process where the transcript is split into 4,000 character. bits that are summarized individually and then concatenated at the end to produce a full summary. And Whisper has a similar issue. Whisper has a limit of 25 megabytes. So your audio has to get chunked too. But once you have this pipeline set up in your organization, it's literally just like drop audio, get summary. And if you wanted to go out and build something like this, this is what it looks like.
Adam:
There are definitely ways to do it. And then SAS competitors doing stuff like this as well. That exists both as APIs and as sort of GUIs that you can use for this kind of stuff. How much of this goes away? That whole chunking process is an attention thing, right?
gptboss:
Yeah, so I've mentioned it a couple times this week, Facebook has a new model that is truly multimodal called ImageBind. And so with that gain of function, with like multimodality gain of function, we don't need a specialized model for transcription. So with more gain of function, like if ImageBind had the intelligence capabilities of GPT-4, it would literally just be like three items. You would... and it had more attention, it would be three items. So the audio file goes in regardless of size, right? Eight, eight hour Slavvoj Cizek lecture marathon, right? That can go in. And then the model transcribes the audio because that's all just tokenizable data. So it takes that audio file in, doesn't even necessarily convert it to text and then starts doing text transformations on the tokens in order to produce the summary at the end. That is, I think, that's a capability that we're going to see before the year's out.
Adam:
And then for example, this, this also includes the part where you go from the audio into the transcript. Um, if you're
gptboss:
You
Adam:
using
gptboss:
wouldn't.
Adam:
a tool like we are like Riverside, you can, you can output transcripts and so you can, you know, there are ways to skip pieces of this that are
gptboss:
Yeah, you don't have
Adam:
manageable
gptboss:
to start with audio.
Adam:
as well. Right. Cool! Well, that's the summaries situation. Maybe we'll come back with more next week and talk about actual sort of use case examples. Or we'll stop dragging summaries out and move on to another topic. Like, subscribe, do all those things and the algorithm will tell you when we're up. Word. OK, let's get out of here. Okay. That's accelerate daily for today. Like I said at the top, if you've got something out of this, like subscribe or even read a review, did I forget to do that at the top?
gptboss:
You got it.
Adam:
Did I?
gptboss:
I don't think you said write a review, but...
Adam:
Yeah, I missed that part. I keep forgetting to do that part because I get lost in the image part. Steve, we're going to have to cut some of this. I'll record it at the end so we can cut it to the beginning of the podcast. Anyway, let's, let's finish the outro though. Okay. That's accelerate daily for today. Like I said, at the top, if you got something out of this, like subscribe or even write a review, those metrics really do make a difference when it comes to reaching more people, uh, working on the future of AI, and if you can make the schedule work, jump in for the live stream, um, you can't, you'll get notified, follow us on socials, LinkedIn, YouTube, all the places, and you'll get notifications when we go live. Uh, and if you can make schedule work, jump in, we watch the chat. Uh, the whole time. So if you have thoughts, you can jump in there. Otherwise, thanks for joining us, everybody. You wanna say something there, Mac?
gptboss:
I just sneezed, sorry. See y'all on
Adam:
Again.
gptboss:
Monday.
Adam:
Say that again so we got it clean.
gptboss:
See you on Monday.
Adam:
Word. Okay, back up and do the intro. Cut in there. Okay, so that's the image of the day. Before we jump into... Before we jump into today's topics, a reminder to like and subscribe wherever you're watching or listening, throwing a comment, write a review, all this really helps us reach the right audience in the algorithms and feeds and everything. It really does make a difference in terms of modern discovery mechanisms and how we find other people, um, other, and, and, and, you know, keep, so we can keep answering questions that the community brings to the table here. Okay. Let's jump into the topics.